Its Not Just Size That Matters: Small Language Models Are Also Few-Shot Learners
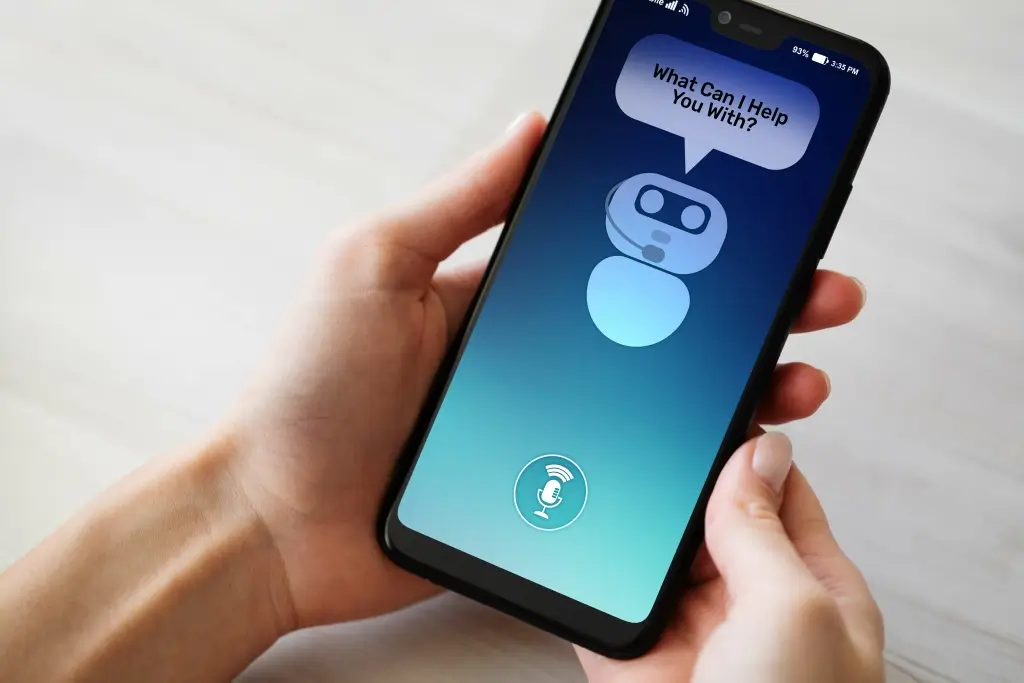
As large language models scale up, they become jacks-of-all-trades but masters of none. What’s more, exposing sensitive data to external LLMs poses security, compliance, and proprietary risks around data leakage or misuse. Up to this point we have covered the general capabilities of small language models and how they confer advantages in efficiency, customization, and oversight compared to massive generalized LLMs. However, SLMs also shine for honing in on specialized use cases by training on niche datasets. How did Microsoft cram a capability potentially similar to GPT-3.5, which has at least 175 billion parameters, into such a small model?
The Rise of Small Language Models – The New Stack
The Rise of Small Language Models.
Posted: Fri, 16 Feb 2024 08:00:00 GMT [source]
For the fine-tuning process, we use about 10,000 question-and-answer pairs generated from the Version 1’s internal documentation. But for evaluation, we selected only questions that are relevant to Version 1 and the process. Further analysis of the results showed that, over 70% are strongly similar to the answers generated by GPT-3.5, that is having similarity 0.5 and above (see Figure 6). In total, there are 605 considered to be acceptable answers, 118 somewhat acceptable answers (below 0.4), and 12 unacceptable answers. Embedding were created for the answers generated by the SLM and GPT-3.5 and the cosine distance was used to determine the similarity of the answers from the two models.
Also, there is a demand for custom Small Language Models that can match the performance of LLMs while lowering the runtime expenses and ensuring a secure and fully manageable environment. These limitations motivate organizations across industries to develop their own small, domain-specific language models using internal data assets. As language models evolve to become more versatile and powerful, it seems that going small may be the best way to go.
Large Language Models: A Leap in the World of Language AI
GPT-3 is the largest language model known at the time with 175 billion parameters trained on 570 gigabytes of text. These models have capabilities ranging from writing a simple essay to generating complex computer codes – all with limited to no supervision. A language model is a statistical and probabilistic tool that determines the probability of a given sequence of words occurring in a sentence. Where weather models predict the 7-day forecast, language models try to find patterns in the human language, one of computer science’s most difficult puzzles as languages are ever-changing and adaptable.
One working group is dedicated to the model’s multilingual character including minority language coverage. To start with, the team has selected eight language families which include English, Chinese, Arabic, Indic (including Hindi and Urdu), and Bantu (including Swahili). Despite all these challenges, very little research is being done to understand how this technology can affect us or how better LLMs can be designed. In fact, the few big companies that have the required resources to train and maintain LLMs refuse or show no interest in investigating them. Facebook has developed its own LLMs for translation and content moderation while Microsoft has exclusively licensed GPT-3. Many startups have also started creating products and services based on these models.
An LLM as a computer file might be hundreds of gigabytes, whereas many SLMs are less than five. Many investigations have found that modern training methods can impart basic language competencies https://chat.openai.com/ in models with just 1–10 million parameters. For example, an 8 million parameter model released in 2023 attained 59% accuracy on the established GLUE natural language understanding benchmark.
As a consequence, for long sequences training times soar because there is no possibility for paralellization. Anthropic Claude — From the makers of ConstitutionalAI focused on model safety, Claude enables easily training custom classifiers, text generators, summarizers, and more with just a few lines of code. Built-in safety constraints and monitoring curb potential risks during deployment. “Most models that run on a local device still need hefty hardware,” says Willison.
From the hardware point of view, it is cheaper to run i.e., SLMs require less computational power and memory and it is suitable for on-premises and on-device deployments making it more secure. In the context of artificial intelligence and natural language processing, SLM can stand for ‘Small Language Model’. The label “small” in this context refers to a) the size of the model’s neural network, b) the number of parameters and c) the volume of data the model is trained on. There are several implementations that can run on a single GPU, and over 5 billion parameters, including Google Gemini Nano, Microsoft’s Orca-2–7b, and Orca-2–13b, Meta’s Llama-2–13b and others. Language model fine-tuning is a process of providing additional training to a pre-trained language model making it more domain or task specific. We are interested in ‘domain-specific fine-tuning’ as it is especially useful when we want the model to understand and generate text relevant to specific industries or use cases.
Microsoft’s 3.8B parameter Phi-3 may rival GPT-3.5, signaling a new era of “small language models.”
One of the main drivers of this change was the emergence of language models as a basis for many applications aiming to distill valuable insights from raw text. The applications above highlight just a snippet of the use cases embracing small language models customized to focused needs. These sorts of customization processes become increasingly arduous for large models. Combined with their accessibility, small language models provide a codex that developers can mold to their particular needs. Phi-3 is immediately available on Microsoft’s cloud service platform Azure, as well as through partnerships with machine learning model platform Hugging Face and Ollama, a framework that allows models to run locally on Macs and PCs.
Most modern language model training leverages some form of transfer learning where models bootstrap capability by first training on broad datasets before specializing to a narrow target domain. The initial pretraining phase exposes models to wide-ranging language examples useful for learning general linguistic rules and patterns. Given the motivations to minimize model size covered above, a natural question arises — how far can we shrink down language models while still maintaining compelling capabilities? Recent research has continued probing the lower bounds of model scale required to complete different language tasks. The smaller model sizes allow small language models to be more efficient, economical, and customizable than their largest counterparts. However, they achieve lower overall capabilities since model capacity in language models has been shown to correlate with size.
In a world where AI has not always been equally available to everyone, they represent its democratization and a future where AI is accessible and tailored to diverse needs. However, because large language models are so immense and complicated, they are often not the best option for more specific tasks. You could use a chainsaw to do so, but in reality, that level of intensity is completely unnecessary. The fine-tuned model seems to competent at extracting and maintaining knowledge while demonstrating the ability to generate answers to the specific domain. A platform agnostic approach allowed us to execute the same fine-tuning processes on AWS and achieve almost identical results without any changes to the code. With a good language model, we can perform extractive or abstractive summarization of texts.
Tiny but mighty: The Phi-3 small language models with big potential – Microsoft
Tiny but mighty: The Phi-3 small language models with big potential.
Posted: Tue, 23 Apr 2024 07:00:00 GMT [source]
Some of the largest language models today, like Google’s PaLM 2, have hundreds of billions of parameters. OpenAI’s GPT-4 is rumored to have over a trillion parameters but spread over eight 220-billion parameter models in a mixture-of-experts configuration. Both models require heavy-duty data center GPUs (and supporting systems) to run properly.
Performance configuration was also enabled for efficient adaptation of pre-trained models. Finally, training arguments were used for defining particulars of the training process and the trainer was passed parameters, data, and constraints. The techniques above have powered rapid progress, but there remain many open questions around how to most effectively train small language models. Identifying the best combinations of model scale, network design, and learning approaches to satisfy project needs will continue keeping researchers and engineers occupied as small language models spread to new domains. Next we’ll highlight some of those applied use cases starting to adopt small language models and customized AI. Large language models require substantial computational resources to train and deploy.

The model that we fine-tuned is Llama-2–13b-chat-hf has only 13 billion parameters while GPT-3.5 has 175 billion. Therefore, due to GPT-3.5 and Llama-2–13b-chat-hf difference in scale, direct comparison between answers was not appropriate, however, small language models the answers must be comparable. It required about 16 hours to complete, and our CPU and RAM resources were not fully utilized during the process. It’s possible that a machine with limited CPU and RAM resources might suit the process.
A 2023 study found that across a variety of domains from reasoning to translation, useful capability thresholds for different tasks were consistently passed once language models hit about 60 million parameters. However, returns diminished after the 200–300 million parameter scale — adding additional capacity only led to incremental performance gains. A single constant running instance of this system will cost approximately $3700/£3000 per month.
We also use fine-tuning methods on Llama-2–13b, a Small Language Model, to address the above-mentioned issues. We are proud to stay that ZIFTM is currently the only
AIOps platform in the market to have a native mobile version! Modern conversational agents or chatbots follow a narrow pre-defined conversational path, while LaMDA can engage in a free-flowing open-ended conversation just like humans.
Not all neural network architectures are equivalently parameter-efficient for language tasks. Careful architecture selection focuses model capacity in areas shown to be critical for language modelling like attention mechanisms while stripping away less essential components. Meanwhile, small language models can readily be trained, deployed, and run on commodity hardware available to many businesses without breaking the bank. Their reasonable resource requirements open up applications in edge computing where they can run offline on lower-powered devices.

Expertise with machine learning itself is helpful but no longer a rigid prerequisite with the right partners. On the flip side, the increased efficiency and agility of SLMs may translate to slightly reduced language processing abilities, depending on the benchmarks the model is being measured against. SLMs find applications in a wide range of sectors, spanning healthcare to technology, and beyond.
Risk management remains imperative in financial services, favoring narrowly-defined language models versus general intelligence. What are the typical hardware requirements for deploying and running Chat PG? One of the key benefits of Small Language Models is their reduced hardware requirements compared to Large Language Models. Typically, SLMs can be run on standard laptop or desktop computers, often requiring only a few gigabytes of RAM and basic GPU acceleration. This makes them much more accessible for deployment in resource-constrained environments, edge devices, or personal computing setups, where the computational and memory demands of large models would be prohibitive. The lightweight nature of SLMs opens up a wider range of real-world applications and democratizes access to advanced language AI capabilities.
It’s estimated that developing GPT-3 cost OpenAI somewhere in the tens of millions of dollars accounting for hardware and engineering costs. Many of today’s publicly available large language models are not yet profitable to run due to their resource requirements. Previously, language models were used for standard NLP tasks, like Part-of-speech (POS) tagging or machine translation with slight modifications. For example, with a little retraining, BERT can be a POS-tagger — because of it’s abstract ability to understand the underlying structure of natural language.
Its researchers found the answer by using carefully curated, high-quality training data they initially pulled from textbooks. “The innovation lies entirely in our dataset for training, a scaled-up version of the one used for phi-2, composed of heavily filtered web data and synthetic data,” writes Microsoft. This smaller size and efficiency is achieved via a few different techniques including knowledge distillation, pruning, and quantization. Knowledge distillation transfers knowledge from a pre-trained LLM to a smaller model, capturing its core capabilities without the full complexity. Pruning removes less useful parts of the model, and quantization reduces the precision of its weights, both of which further reduce its size and resource requirements. Please note that we used GPT-3.5 to generate questions and answers from the training data.
Like we mentioned above, there are some tradeoffs to consider when opting for a small language model over a large one. Overall, despite the initial challenges of understanding the interconnections and facing several unsuccessful attempts, the fine-tuning process appeared to run smoothly and consistently. However, this cost above did not include the cost of all trials and errors that concluded to the final fine-tuning process. An improvement regarding this matter is the use of Recurrent Neural Networks (RNNs) (if you’d like a thorough explanation of RNNs I suggest reading this article). Being either an LSTM or a GRU cell based network, it takes all previous words into account when choosing the next word. For a further explanation on how RNNs achieve long memory please refer to this article.
Some popular SLM architectures include distilled versions of GPT, BERT, or T5, as well as models like Mistral’s 7B, Microsoft’s Phi-2, and Google’s Gemma. These architectures are designed to balance performance, efficiency, and accessibility. As far as use cases go, small language models are often used in applications like chatbots, virtual assistants, and text analytics tools deployed in resource-constrained environments.
Moreover, the language model is practically a function (as all neural networks are, with lots of matrix computations), so it is not necessary to store all n-gram counts to produce the probability distribution of the next word. 🤗 Hugging Face Hub — Hugging Face provides a unified machine learning ops platform for hosting datasets, orchestrating model training pipelines, and efficient deployment for predictions via APIs or apps. Their Clara Train product specializes in state-of-the-art self-supervised learning for creating compact yet capable small language models.
Large language models have been top of mind since OpenAI’s launch of ChatGPT in November 2022. From LLaMA to Claude 3 to Command-R and more, companies have been releasing their own rivals to GPT-4, OpenAI’s latest large multimodal model. The quality and feasibility of your dataset significantly impact the performance of the fine-tuned model. For our goal in this phase, we need to extract text from PDF’s, to clean and prepare the text, then we generate question and answers pairs from the given text chunks. This one-year-long research (from May 2021 to May 2022) called the ‘Summer of Language Models 21’ (in short ‘BigScience’) has more than 500 researchers from around the world working together on a volunteer basis. The services above exemplify the turnkey experience now realizable for companies ready to explore language AI’s possibilities.
Relative to baseline Transformer models, Efficient Transformers achieve similar language task performance with over 80% fewer parameters. Effective architecture decisions amplify the ability companies can extract from small language models of limited scale. Small language models can capture much of this broad competency during pretraining despite having limited parameter budgets. Specialization phases then afford refinement towards specific applications without needing to expand model scale.
Small language models are essentially more streamlined versions of LLMs, in regards to the size of their neural networks, and simpler architectures. Compared to LLMs, SLMs have fewer parameters and don’t need as much data and time to be trained — think minutes or a few hours of training time, versus many hours to even days to train a LLM. Because of their smaller size, SLMs are therefore generally more efficient and more straightforward to implement on-site, or on smaller devices. They are gaining popularity and relevance in various applications especially with regards to sustainability and amount of data needed for training.
With attentiveness to responsible development principles, small language models have potential to transform a great number of industries for the better in the years ahead. We’re just beginning to glimpse the possibilities as specialized AI comes within reach. Entertainment’s creative latitude provides an ideal testbed for exploring small language models generative frontiers.
Our GPU usage aligns with the stated model requirements; perhaps increasing the batch size could accelerate the training process. First, the LLMs are bigger in size and have undergone more widespread training when weighed with SLMs. Second, the LLMs have notable natural language processing abilities, making it possible to capture complicated patterns and outdo in natural language tasks, for example complex reasoning.

Their simple web interface masks infrastructure complexity for model creation and monitoring. Transfer learning training often utilizes self-supervised objectives where models develop foundational language skills by predicting masked or corrupted portions of input text sequences. These self-supervised prediction tasks serve as pretraining for downstream applications. According to Microsoft, the efficiency of the transformer-based Phi-2 makes it an ideal choice for researchers who want to improve safety, interpretability and ethical development of AI models. The science of extracting information from textual data has changed dramatically over the past decade. As the term Natural Language Processing took over Text Mining as the name of this field, the methodology used has changed tremendously, too.
A simple probabilistic language model (a) is constructed by calculating n-gram probabilities (an n-gram being an n word sequence, n being an integer greater than 0). An n-gram’s probability is the conditional probability that the n-gram’s last word follows the a particular n-1 gram (leaving out the last word). Practically, it is the proportion of occurences of the last word following the n-1 gram leaving the last word out. This concept is a Markov assumption — given the n-1 gram (the present), the n-gram probabilities (future) does not depend on the n-2, n-3, etc grams (past) .
Recently, small language models have emerged as an interesting and more accessible alternative to their larger counterparts. In this blog post, we will walk you through what small language models are, how they work, the benefits and drawbacks of using them, as well as some examples of common use cases. These issues might be one of the many that are behind the recent rise of small language models or SLMs. The collaborative is divided into multiple working groups, each investigating different aspects of model development. One of the groups will work on calculating the model’s environmental impact, while another will focus on responsible ways of sourcing the training data, free from toxic language.
Benefits and Drawbacks of Small Language Models
AllenNLP’s ELMo takes this notion futher by utilising a bidirectional LSTM, thereby all context before and after the word counts. Both individuals and organizations that work with arXivLabs have embraced and accepted our values of openness, community, excellence, and user data privacy. ArXiv is committed to these values and only works with partners that adhere to them. Financial corporations also deploy SLMs for needs around analyzing earnings statements, asset valuations, risk modeling and more. Community created roadmaps, articles, resources and journeys for
developers to help you choose your path and grow in your career.
- One of the key benefits of Small Language Models is their reduced hardware requirements compared to Large Language Models.
- We are proud to stay that ZIFTM is currently the only
AIOps platform in the market to have a native mobile version!
- A 2023 study found that across a variety of domains from reasoning to translation, useful capability thresholds for different tasks were consistently passed once language models hit about 60 million parameters.
- However, while the capabilities of LLMs are impressive, their massive size leads to downsides in efficiency, cost, and customizability.
- These limitations motivate organizations across industries to develop their own small, domain-specific language models using internal data assets.
Secondly, the goal was to create an architecture that gives the model the ability to learn which context words are more important than others. Neural network based language models (b) ease the sparsity problem by the way they encode inputs. Embedding layers create an arbitrary sized vector of each word that incorporates semantic relationships as well (if you are not familiar with word embeddings, I suggest reading this article). These continous vectors create the much needed granularity in the probability distribution of the next word.
Over the past few year, we have seen an explosion in artificial intelligence capabilities, much of which has been driven by advances in large language models (LLMs). Models like GPT-3, which contains 175 billion parameters, have shown the ability to generate human-like text, answer questions, summarize documents, and more. However, while the capabilities of LLMs are impressive, their massive size leads to downsides in efficiency, cost, and customizability. This has opened the door for an emerging class of models called Small Language Models (SLMs). For example, Efficient Transformers have become a popular small language model architecture employing various techniques like knowledge distillation during training to improve efficiency.
- Overall, transfer learning greatly improves data efficiency in training small language models.
- In fairness, transfer learning shines in the field of computer vision too, and the notion of transfer learning is essential for an AI system.
- Most modern language model training leverages some form of transfer learning where models bootstrap capability by first training on broad datasets before specializing to a narrow target domain.
- Many investigations have found that modern training methods can impart basic language competencies in models with just 1–10 million parameters.
- We are interested in ‘domain-specific fine-tuning’ as it is especially useful when we want the model to understand and generate text relevant to specific industries or use cases.
- Thanks to their smaller codebases, the relative simplicity of SLMs also reduces their vulnerability to malicious attacks by minimizing potential surfaces for security breaches.
The impressive power of large language models (LLMs) has evolved substantially during the last couple of years. While Small Language Models and Transfer Learning are both techniques to make language models more accessible and efficient, they differ in their approach. SLMs can often outperform transfer learning approaches for narrow, domain-specific applications due to their enhanced focus and efficiency. Parameters are numerical values in a neural network that determine how the language model processes and generates text. They are learned during training on large datasets and essentially encode the model’s knowledge into quantified form. More parameters generally allow the model to capture more nuanced and complex language-generation capabilities but also require more computational resources to train and run.
Overall there’s greater potential to find profitable applications of small language models in the short-term. ✨ Cohere for AI — Cohere offers a developer-friendly platform for building language models down to 1 million parameters drawing from their own training data or imported custom sets. You can foun additiona information about ai customer service and artificial intelligence and NLP. Of course, specialized small language models tuned deeply rather than broadly may require much less capacity to excel at niche tasks. But first, let’s overview popular techniques for effectively training compact yet capable small language models. A key advantage that small language models maintain over their largest counterparts is customizability. While models like GPT-3 demonstrate strong versatility across many tasks, their capabilities still represent a compromise solution that balances performance across domains.
The experiential technology of small language models distills broad excitement around language AI down to practical building blocks deliverable in the hands of commercial teams and users. Still an industry in its infancy, unlocking new applications harnesses both developer creativity and thoughtfulness on impacts as specialized models spread. But tailorable language intelligence now arriving on the scene appears poised to drive the next phase of AI productivity. These applications translate language AI into direct process automation and improved analytics within established financial workflows — accelerating profitable models rather than speculating on technology promises alone.
On Tuesday, Microsoft announced a new, freely available lightweight AI language model named Phi-3-mini, which is simpler and less expensive to operate than traditional large language models (LLMs) like OpenAI’s GPT-4 Turbo. Its small size is ideal for running locally, which could bring an AI model of similar capability to the free version of ChatGPT to a smartphone without needing an Internet connection to run it. Small Language Models often utilize architectures like Transformer, LSTM, or Recurrent Neural Networks, but with a significantly reduced number of parameters compared to Large Language Models.